TABLE OF CONTENTS
Experience the Future of Speech Recognition Today
Try Vatis now, no credit card required.
OpenAI Whisper is a groundbreaking automatic speech recognition technology that converts spoken language into written text with impressive accuracy and versatility. Since its release in September 2022, Whisper has quickly gained recognition for its ability to handle diverse speech patterns, languages, and environments, making it a preferred choice for developers and researchers alike. In this blog post, we will take a deep dive into Whisper’s underlying technology, discuss its unique capabilities, and examine its advantages and limitations. We'll also explore alternatives and provide guidance on selecting the best speech-to-text solution for your needs.
Why OpenAI Whisper? The Evolution of Speech-to-Text Systems
Before Whisper’s release, ASR technology faced several challenges, including difficulty handling diverse languages, managing noisy environments, and achieving accurate transcription for low-resource languages. Many existing systems struggled to balance speed, accuracy, and adaptability. OpenAI Whisper was designed to address these challenges head-on with a transformer-based architecture and a massive, diverse training dataset. It aims to provide a versatile, high-accuracy speech-to-text solution capable of understanding nuanced speech patterns across different contexts and languages.
Understanding Whisper's Architecture
The Whisper architecture employs a straightforward end-to-end approach using an encoder-decoder Transformer. Audio input is divided into 30-second segments, which are converted into log-Mel spectrograms and fed into the encoder. The decoder is trained to generate the corresponding text captions, incorporating special tokens that guide the model in performing various tasks, such as language identification, phrase-level timestamping, multilingual speech transcription, and speech translation into English.
This section explains the architecture in greater detail:
Encoder: The input audio is split into 30-second chunks and converted into a log-Mel spectrogram, a visual representation that captures the frequency content of the audio signal over time. This spectrogram serves as a mathematical representation of the audio data. The encoder processes this spectrogram to extract underlying patterns, features, and characteristics within the speech, including tone, pitch, and duration.
Decoder: The decoder leverages a sophisticated language model to interpret the encoded representation from the encoder. It uses the context provided by the audio data to predict the most likely sequence of text tokens (the basic units of text used for processing). This prediction generates the final transcript, balancing language understanding and context awareness.
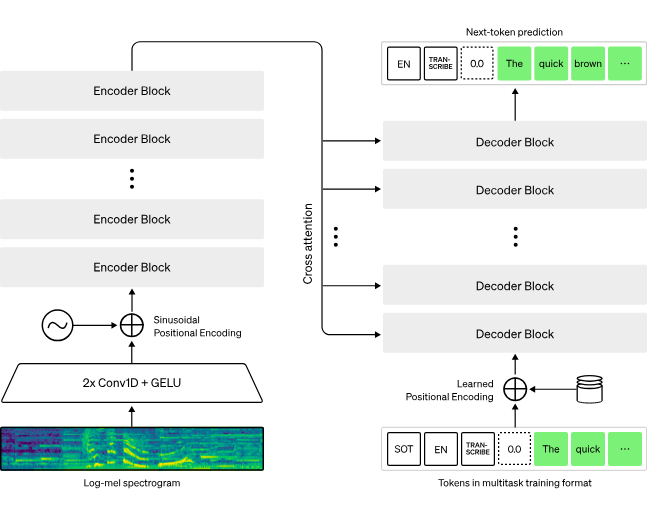
Source: OpenAI

Source: OpenAI
Whisper's architecture stands out due to its ability to handle long-range dependencies within speech, ensuring accurate transcription of diverse speech patterns. Its transformer-based design allows it to process multiple audio features simultaneously, making it highly effective in varied scenarios.
The Role of Training Data: Scope and Diversity
A critical factor contributing to Whisper's success is its extensive training dataset, which consists of over 680,000 hours of supervised speech data. This vast dataset encompasses a wide range of languages, accents, dialects, and audio environments.
Here’s why this matters:
Diversity: The dataset includes various types of audio data, such as conversational speech, news broadcasts, podcasts, and more. This diversity allows Whisper to generalize effectively across different contexts and applications.
Exposure to Rare Languages: By training on a large dataset that includes low-resource languages, Whisper can recognize and transcribe speech in 99 languages, offering robust support for global users.
Adaptability to Different Environments: The dataset includes recordings with various background noises, accents, and speaking styles, enhancing Whisper's robustness in real-world situations.
However, training on such a large and diverse dataset also raises potential concerns about bias and representation, which are important considerations when deploying Whisper in sensitive or high-stakes applications.
Performance Metrics: What Makes Whisper Accurate?
Whisper's accuracy is often highlighted through key performance metrics:
- Word Error Rate (WER): Whisper consistently demonstrates a low WER across multiple languages and contexts, making it a reliable choice for applications where transcription quality is paramount.
- Handling of Diverse Speech Patterns: Whisper is particularly effective in managing homophones, accents, and domain-specific jargon, often outperforming other ASR systems in multilingual or low-resource scenarios.
Comparing Whisper to Other Open-Source ASR Systems
There are many ways to accuracy test speech-to-text models, however a common way is to compare models against highly curated audio datasets, so that it's a fair comparison. For this comparison, we will compare the top open-source speech-to-text softwares against each other using the Common Voice and LibriSpeech datasets.
Common Voice
Common Voice is an amazing initiative from Mozilla to curate voices from people across the internet. Anyone can contribute to the project either by providing a voice snippet, or listening to existing voice snippets and providing feedback on the transcription quality. It is truly an experience curated by the community at large, which is exciting.
LibriSpeech
LibriSpeech is a common audio dataset derived from read audiobooks, carefully segmented and aligned to ensure accuracy of the dataset.
Here's a summary of the latest benchmarks comparing Word Error Rate (WER) performance across various open-source automatic Speech Recognition (ASR) systems.
Whisper (OpenAI)
- Common Voice Dataset: 9.0% WER
- LibriSpeech Dataset: 2.7% WER (clean) and 5.2% WER (other)
- Whisper generally performs well across both datasets, demonstrating superior accuracy, especially in noisy conditions (WhisperAPI
Mozilla DeepSpeech
- Common Voice Dataset: 43.82% WER
- LibriSpeech Dataset: 7.27% WER (clean) and 21.45% WER (other)
- DeepSpeech show s significantly higher error rates compared to Whisper, especially in challenging datasets (WhisperAPI)
Kaldi
- Common Voice Dataset: 4.44% WER
- LibriSpeech Dataset: 3.8% WER (clean) and 8.76% WER (other)
- Kaldi shows competitive performance, particularly on the LibriSpeech dataset. However, it involves a more complex setup and usage compared to Whisper(WhisperAPI)
Wav2vec 2.0 (Facebook AI)
- Common Voice Dataset: 16.1% WER (English only)
- LibriSpeech Dataset: 2.2% WER (clean) and 4% WER (other)
- wav2vec 2.0 shows excellent performance on the LibriSpeech dataset, outperforming Whisper in specific scenarios, particularly in clean environments (WhisperAPI)
NeMo (NVIDIA)
- Common Voice Dataset: 7.5% WER (English only)
- LibriSpeech Dataset: 1.8% WER (clean) and 3.3% WER (other)
- NeMo demonstrates strong performance, especially on the LibriSpeech dataset, slightly outperforming Whisper in clean environments. NeMo is particularly well-suited for real-time applications due to its optimization for multi-GPU training and inference.

Source: Whisper, Kaldi, Mozilla DeepSpeech, wav2vec 2.0 WER Data: Accuracy Benchmarks of The Top Free Open Source Speech-to-Text Offerings from Whisper API
Conclusion:
Whisper generally offers a strong balance of accuracy and versatility, with particularly strong performance in multilingual transcription and noisy environments.
However, for specific tasks, other open-source ASR models like NeMo, Kaldi or Wav2vec 2.0 might offer competitive or even superior performance.
Key Capabilities of Whisper
- Speech-to-Text Transcription: Converts spoken language into written text, handling various audio formats, noise levels, and speaking styles.
- Multilingual Speech Recognition: Supports transcription in 99 languages, including many low-resource languages.
- Translation: Can translate speech from any of its supported languages into English text.
- Customizability: Allows fine-tuning to enhance performance for specific domains, languages, and accents.
Practical Use Cases and Applications
Whisper's versatility makes it suitable for various industries and applications:
- Healthcare: Accurately transcribing medical dictations and patient interactions to reduce administrative workload and improve documentation accuracy.
- Media and Entertainment: Generating multilingual subtitles for videos and podcasts, enabling content accessibility across different languages.
- Customer Service: Real-time transcription in call centers, supporting multilingual customer interactions and improving response times.
- Education: Assisting in language learning and accessibility by providing accurate transcriptions and translations of lectures or course materials.
Factors Contributing to Whisper's Success
- Large Training Dataset: Ensures exposure to diverse audio characteristics and speaking styles, contributing to its high accuracy.
- Transformer Architecture: Captures long-range dependencies within speech, leading to more precise transcriptions.
- Adaptability: Fine-tuning capabilities make Whisper suitable for specific use cases and industries, enhancing its utility and performance.
Limitations to Consider When Using Whisper
While Whisper offers significant advantages, it is essential to be aware of its limitations:
- Scalability Challenges: The open-source version of Whisper has limitations on file size (25MB) and audio duration (30 seconds). Additionally, it lacks support for features like real-time transcription and speaker diarization.
- Accuracy Trade-offs: Prioritizes accuracy over speed. For applications requiring faster processing, smaller Whisper models or alternative ASR systems might be better suited.
- In-House Expertise Required: Deploying and maintaining Whisper at scale necessitates in-house AI expertise for customization and hardware management.
Alternatives to OpenAI Whisper
If Whisper’s limitations do not align with your needs, several alternatives offer different functionalities and performance characteristics:
Open-Source Alternatives:
- Mozilla DeepSpeech: Enables training custom models for specific needs.
- Kaldi: A powerful toolkit for speech recognition systems with extensive customization options.
- Wav2Vec: Meta AI's speech recognition system known for high-performing speech processing.
- NVIDIA NeMo: Offers pre-trained models, optimized for real-time performance, and supports multi-GPU training, making it suitable for both research and production use cases
Commercial Alternatives:
- Big Tech Cloud Services: Google Cloud Speech-to-Text, Microsoft Azure AI Speech Services, and Amazon Transcribe provide multilingual speech-to-text functionalities.
- Specialized Speech-to-Text APIs: companies like Vatis Tech, AssemblyAI, and Deepgram excel in domain-specific applications, ensuring high-quality transcriptions with real-world relevance and customization options for target customers. They often offer additional features like speaker diarization or sentiment analysis.